- Harbourfront Quantitative Newsletter
- Posts
- Machine Learning in Financial Markets: When It Works and When It Doesn’t
Machine Learning in Financial Markets: When It Works and When It Doesn’t
How to identify when machine learning models add real value
Machine learning (ML) has made a lot of progress in recent years. However, there are still skeptics, especially when it comes to its application in finance. In this issue, I will feature articles that discuss the pros and cons of ML. In future editions, I’ll explore specific techniques.
In this issue:
Latest Posts
Do Calendar Anomalies Still Work? Evidence and Strategies (11 min)
Catastrophe Bonds: Modeling Rare Events and Pricing Risk (10 min)
Breaking Down Volatility: Diffusive vs. Jump Components (10 min)
Crypto Market Arbitrage: Profitability and Risk Management (10 min)
Optimizing Portfolios: Simple vs. Sophisticated Allocation Strategies (10 min)
Big Tech Has Spent Billions Acquiring AI Smart Home Startups
The pattern is clear: when innovative companies successfully integrate AI into everyday products, tech giants pay billions to acquire them.
Google paid $3.2B for Nest.
Amazon spent $1.2B on Ring.
Generac spent $770M on EcoBee.
Now, a new AI-powered smart home company is following their exact path to acquisition—but is still available to everyday investors at just $1.90 per share.
With proprietary technology that connects window coverings to all major AI ecosystems, this startup has achieved what big tech wants most: seamless AI integration into daily home life.
Over 10 patents, 200% year-over-year growth, and a forecast to 5x revenue this year — this company is moving fast to seize the smart home opportunity.
The acquisition pattern is predictable. The opportunity to get in before it happens is not.
Past performance is not indicative of future results. Email may contain forward-looking statements. See US Offering for details. Informational purposes only.
How Accurate is Machine Learning Prediction in Finance?
Machine Learning has many applications in finance, such as predicting stock prices, detecting fraudulent activities, and automating investment decisions. However, the accuracy of ML prediction can vary widely depending on the type of data used and the model chosen.
Reference [1] discusses the problems that Machine Learning is facing in finance.
Findings
Recent research suggests that machine learning (ML) is valuable in asset pricing due to its ability to capture nonlinearities and interaction effects that traditional models often miss.
Machine learning is highly effective for applications with large datasets and high signal-to-noise ratios, but financial market data often lacks these characteristics.
Financial markets evolve over time, meaning anomalies detected by ML can be arbitraged away, rendering past data less relevant for future predictions.
An analogy highlights the challenge: once an ML algorithm learns to recognize "cats" in an image, all "cats" could morph into "dogs," requiring the algorithm to relearn from scratch.
There is a risk of positive publication bias, overfitting, and reliance on the assumption that past relationships will persist in the future.
Human expertise remains crucial due to the low signal-to-noise ratio in financial data and the limitations of ML models.
In summary, the paper concludes that while ML does show promise, its superior performance is often overstated. When practical challenges are taken into account, the performance gap between ML and traditional methods narrows. However, investors who follow a rigorous and disciplined research process can still benefit meaningfully from ML-based strategies.
Reference
[1] Blitz, David and Hoogteijling, Tobias and Lohre, Harald and Messow, Philip, How Can Machine Learning Advance Quantitative Asset Management? (2025), SSRN 4321398
Machine Learning: Is More Data Always Better?
Reference [2] discusses the question whether more data is always beneficial in machine learning.
Findings
The paper delves into the nuanced aspect of data quantity, questioning the assumption that more data necessarily leads to better machine learning outcomes.
It argues that older data may lose relevance over time and including it can actually reduce model accuracy.
Increasing the flow of data, or collecting data at a higher rate, tends to improve model accuracy but requires more frequent model retraining.
Quality vs. Quantity: It discusses the trade-off between the quality and quantity of data, suggesting that the relevance and quality of the data are crucial factors in the effectiveness of machine learning models.
The business value of machine learning models does not necessarily scale with the amount of stored data, especially if the data becomes outdated.
Firms should adopt a growth policy that balances the retention of historical data with the acquisition of fresh data.
Real-world Applications: Examples from various industries, such as healthcare, are presented to illustrate scenarios where the volume of data may not be the sole determinant of success in machine learning applications.
What implication does this paper have for trading and portfolio management? Should we use more data?
The short answer is probably no. In fact, using more data can actually lead to sub-optimal results. The reason is that, in the financial world, data is often noisy and contains a lot of irrelevant information. If you use too much data, your machine learning models will end up picking up on this noise, which can lead to sub-optimal results.
Reference
[2] Valavi, Ehsan, Joel Hestness, Newsha Ardalani, and Marco Iansiti. Time and the Value of Data. Harvard Business School Working Paper, No. 21-016
Closing Thoughts
In this issue, I discussed the advantages and disadvantages of machine learning techniques as applied in finance. However, as the field is progressing rapidly, many of the current limitations, such as overfitting, interpretability, and data relevance, are being actively addressed by researchers and practitioners. With a disciplined research process and model design, investors can harness the strengths of machine learning to enhance forecasting, risk management, and strategy development.
Educational Video
Why Every Trader Needs to Know This: Dr. Thomas Starke on Machine Learning Trading
In this video, Dr. Thomas Starke explores the transformative potential of Deep Reinforcement Learning (DRL) in financial markets. He explains how DRL, the same technology that mastered the world’s most complex board game, Go, is now poised to revolutionize algorithmic trading.
Dr. Starke walks through key concepts such as Markov Decision Processes and their application to trading, the Bellman Equation, and the complexities involved in training and testing DRL models.
Volatility Weekly Recap
The figure below shows the term structures for the VIX futures (in colour) and the spot VIX (in grey).
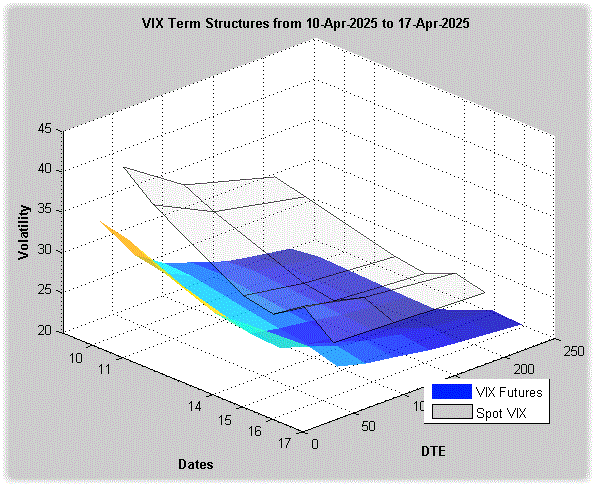
Stocks wrapped up another volatile week with a mixed day, as the global trade war remains top of mind and tensions rose between the Trump administration and the Fed. The Dow ended 527 points down, with stocks booking weekly losses ahead of the long weekend. Despite the loss in the S&P 500, the VIX index ended lower and finished the week below 30. VIX futures also finished the week lower compared to last week; however, they still remain in backwardation. This resulted in returns of -7.49% and -0.63% for VXX and VIXM respectively.

Around the Quantosphere
Zero-day options are fueling the unprecedented volatility on Wall Street amid tariff chaos (cnbc)
Top Trends in Quantitative Finance to Watch in 2025 (streetinsider)
From enormous payouts to pink slips: What it's like to work at a hedge fund right now (businessinsider)
Quant researcher at Citadel Securities: "I spent 4 years studying day and night" (efinancialcareers)
Podcast: Eugene Fama and David Booth on the Birth of Modern Finance (bloomberg)
Disclaimer
This newsletter is not investment advice. It is provided solely for entertainment and educational purposes. Always consult a financial professional before making any investment decisions.
We are not responsible for any outcomes arising from the use of the content and codes provided in the outbound links. By continuing to read this newsletter, you acknowledge and agree to this disclaimer.